Artificial Intelligence (AI) is transforming industries and reshaping the way organizations operate, offering unprecedented opportunities for automation, decision-making, and efficiency. However, the rapid adoption of AI technologies also brings forth significant security challenges that must be addressed to mitigate risks and foster trust in AI-driven solutions.
The Importance of AI Security
AI Security encompasses a range of practices and methodologies aimed at protecting AI systems, data, and applications from potential threats and vulnerabilities. As AI becomes increasingly integrated into critical business processes and consumer-facing applications, ensuring the confidentiality, integrity, and availability of AI-driven insights and operations becomes paramount.
Securing AI Models and Data
One of the primary concerns in AI security is safeguarding AI models and the data used to train them. Organizations must implement robust data governance frameworks to ensure data privacy and compliance with regulatory requirements. Techniques such as encryption and secure data storage methodologies protect sensitive data from unauthorized access and breaches, preserving the integrity of AI models and the trustworthiness of AI-driven decisions.
Mitigating Adversarial Attacks
Adversarial attacks pose a significant threat to AI systems, exploiting vulnerabilities in AI algorithms to manipulate outputs or compromise system integrity. Techniques such as adversarial training, which involves exposing AI models to adversarial examples during the training phase, help improve resilience against such attacks. Continuous monitoring and anomaly detection mechanisms further enhance the ability to detect and mitigate adversarial threats in real-time.
Ensuring Robust Authentication and Access Control
Authentication and access control mechanisms are critical in securing AI systems and preventing unauthorized access. Multi-factor authentication (MFA), strong password policies, and role-based access controls (RBAC) limit access to AI models, data, and infrastructure based on the principle of least privilege. Implementing these measures ensures that only authorized users and applications can interact with AI systems, reducing the risk of insider threats and unauthorized data manipulation.
Integrating Security into AI Development Lifecycle
Embedding security into the AI development lifecycle is essential for building resilient and trustworthy AI systems. Security considerations should be integrated from the initial design phase through development, testing, deployment, and maintenance. Adopting secure coding practices, conducting rigorous security testing, and performing regular security audits help identify and address vulnerabilities early, minimizing security risks throughout the AI lifecycle.
Regulatory Compliance and Ethical Considerations
Adherence to regulatory requirements and ethical guidelines is crucial in AI security. Organizations must comply with data protection regulations such as GDPR, HIPAA, and others relevant to their industry. Ethical considerations, including fairness, transparency, and accountability in AI decision-making processes, ensure responsible AI deployment and mitigate risks associated with bias and discrimination.
Collaboration and Knowledge Sharing
Effective AI security requires collaboration among stakeholders, including AI developers, security experts, regulators, and policymakers. Sharing threat intelligence, best practices, and lessons learned fosters collective defense capabilities and enhances resilience against evolving cyber threats targeting AI systems.
Looking Ahead: Future Challenges and Opportunities
As AI continues to advance, new challenges and opportunities in AI security will emerge. Addressing challenges such as explainability, robustness, and scalability of AI security solutions requires ongoing innovation, research, and collaboration across disciplines. Embracing emerging technologies like AI-driven security analytics and autonomous threat detection enables organizations to stay ahead of evolving threats and maintain trust in AI-driven innovations.
Conclusion
In conclusion, AI security is essential for harnessing the transformative potential of AI while mitigating associated risks. By implementing robust security measures, integrating security into the AI development lifecycle, adhering to regulatory requirements, and promoting ethical AI practices, organizations can build resilient AI systems that enhance productivity, innovation, and trust in an interconnected and AI-driven world. Embracing a proactive approach to AI security ensures a secure foundation for leveraging AI’s capabilities while safeguarding data, privacy, and organizational reputation.
Ensuring AI Security: Safeguarding Innovation in a Connected World
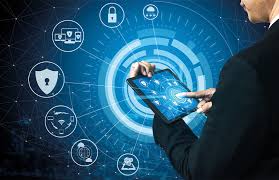
Categories: